How AI and Machine Learning Are Revolutionizing Drug Discovery
- Issa Kildani
- Oct 22, 2024
- 5 min read
The pharmaceutical industry is witnessing a transformative shift in how drugs are discovered, developed, and marketed. Artificial intelligence (AI) and machine learning (ML) are at the forefront of this revolution, reshaping the drug discovery process. Traditionally, drug discovery has been a long, complex, and costly endeavor, but with AI, this landscape is changing rapidly. In 2024, AI is unlocking new possibilities for faster drug discovery, reducing costs, and increasing the accuracy of predictions in preclinical and clinical research.

The Traditional Drug Discovery Process: A Bottleneck of Time and Cost
Drug discovery typically involves several stages: target identification, lead discovery, preclinical studies, clinical trials, and regulatory approval. This process can take over a decade and cost billions of dollars, often with high failure rates. Only a fraction of thousands of screened compounds make it to human testing, and fewer still reach the market.
A Tufts Center for the Study of Drug Development study estimates that the average cost to bring a drug to market is approximately $2.6 billion. Moreover, more than 90% of drugs that enter clinical trials never make it to approval. This inefficiency stems from a complex interplay of factors, including unpredictable biological responses, challenges in predicting human drug reactions based on animal models, and the time-consuming nature of manual data analysis.
AI and Machine Learning: Speeding Up Target Identification
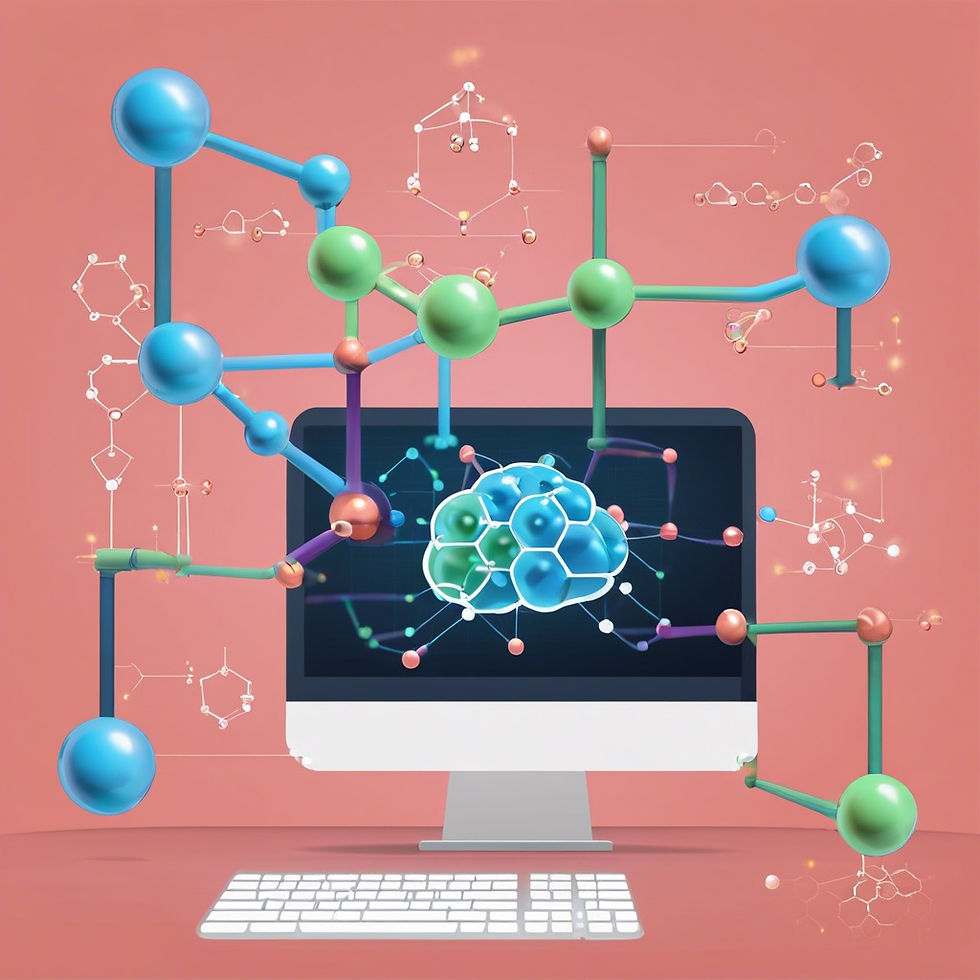
AI and machine learning are critical tools in transforming the target identification phase of drug discovery. Traditionally, identifying potential biological targets involves years of laboratory experiments and genetic research. AI, however, can analyze vast amounts of biological and chemical data at unprecedented speeds, making predictions and identifying patterns that would be difficult for humans to recognize.
AI models can rapidly analyze genomic data, proteomics, and disease pathways, allowing researchers to identify potential drug targets more efficiently. For instance, deep learning models can be trained to recognize specific genetic mutations or molecular interactions associated with certain diseases, accelerating the process of pinpointing druggable targets. A company like Insilico Medicine has made waves by using AI to identify novel targets for cancer and age-related diseases. These models not only speed up the identification process but also improve the likelihood of success by suggesting targets with higher therapeutic potential.
Predictive Models and Virtual Screening: Enhancing Lead Discovery
Once a target is identified, the next step is discovering a lead compound that can modulate this target's activity. Lead discovery traditionally relied on high-throughput screening (HTS), where researchers test thousands of compounds in vitro (in test tubes) to identify candidates for further testing. However, this method is time-consuming and resource-intensive and often yields low success rates.

AI and ML are transforming this phase through virtual screening and predictive models. By leveraging large datasets of molecular structures and bioactivity data, machine learning models can predict the interactions between chemical compounds and biological targets. These models analyze chemical libraries of millions of compounds in a fraction of the time it would take to conduct physical experiments. Predictive models powered by AI, such as those developed by companies like Atomwise, can suggest which compounds are most likely to succeed in modulating a target, reducing the number of compounds that need to be physically tested.
This computational approach, often called in silico drug discovery, allows researchers to test various molecular interactions and refine compounds more accurately. In some cases, AI has identified viable drug candidates within months—compared to years using traditional methods.
AI's Role in Preclinical Studies and Drug Design
Drug design has also benefited from AI-driven innovation. Preclinical studies, where drugs are tested on cell cultures or animal models, are necessary to establish a compound's efficacy and safety. AI helps optimize drug design by predicting how molecular structures will behave in biological systems, allowing for better-informed decisions before advancing compounds into costly animal or human trials.
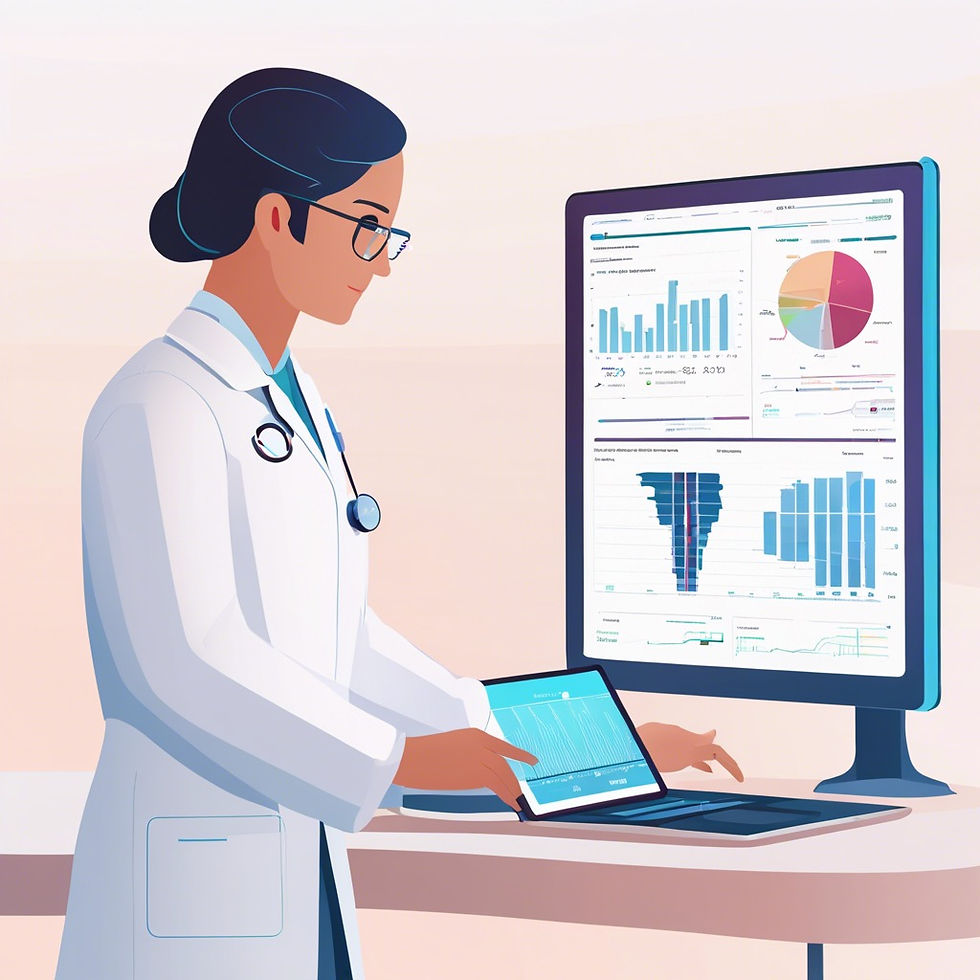
Generative adversarial networks (GANs) and reinforcement learning are being applied to create novel chemical entities with desired properties. These AI models can generate new compounds that fit a specific drug target profile, simulating chemical reactions and optimizing pharmacokinetics (absorption, distribution, metabolism, and excretion of the drug) and pharmacodynamics (the drug's biological effects).
. The iterative process between virtual screening and optimization allows for the rapid creation of molecules with a higher chance of success.
AI-Driven Clinical Trials: Improving Efficiency and Patient Selection
Clinical trials represent the most expensive and time-consuming stage of drug development. Many drugs fail in this phase due to poor efficacy, unanticipated side effects, or difficulty recruiting suitable patients. AI has the potential to revolutionize clinical trials by improving patient recruitment, optimizing trial design, and enabling real-time data analysis.
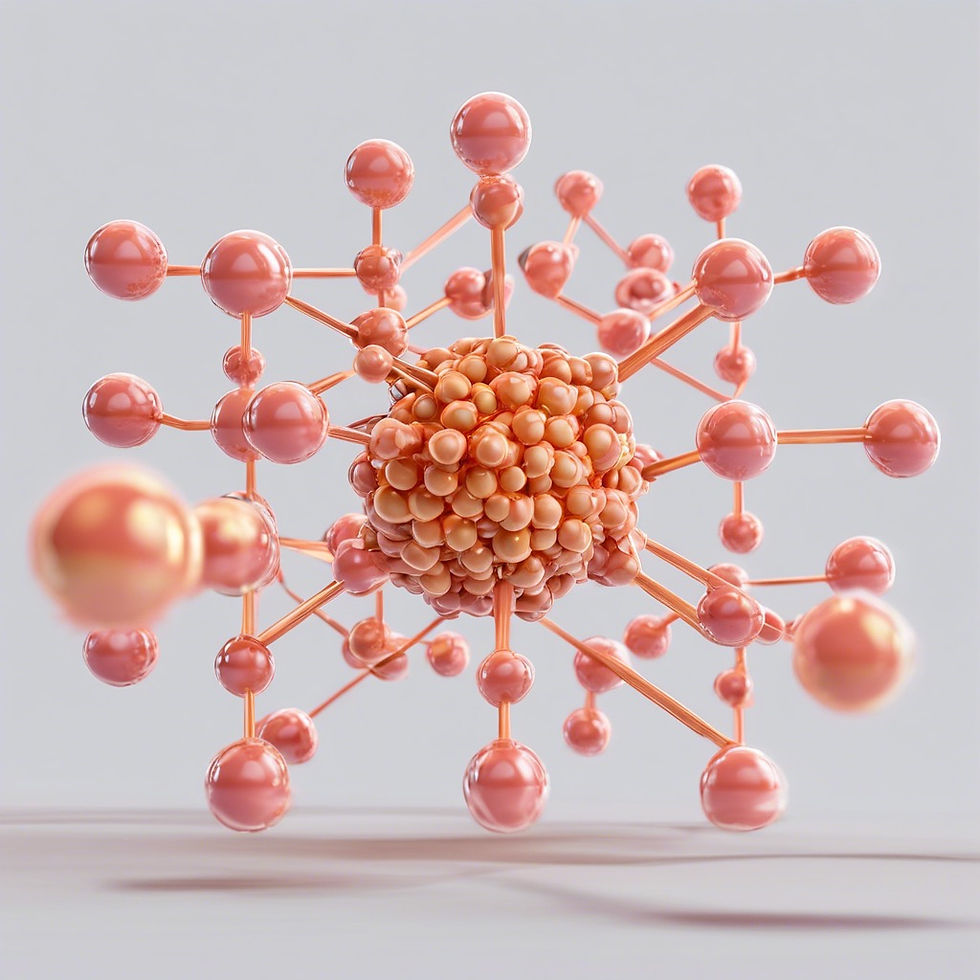
One of the key challenges in clinical trials is identifying the right patients. AI algorithms, powered by vast datasets from electronic health records (EHRs), genetic profiles, and patient history, can better match patients to trials than traditional methods. Companies like IBM Watson Health use AI to analyze genomic data and predict which patients will respond best to specific therapies, leading to more personalized treatment approaches. This has been particularly transformative in oncology, where genetic mutations play a significant role in determining a patient's response to drugs.
AI can also assist in adaptive trial designs, where trial protocols are modified in real-time based on incoming data. For example, suppose a drug shows promising results in a subset of patients. In that case, the trial can be adjusted to focus on those patients, potentially speeding up the process of getting effective treatments to market. AI-driven data analytics enable real-time monitoring of trial data, ensuring early detection of adverse effects or efficacy signals.
.
Ethical Considerations and Challenges in AI-Driven Drug Discovery
While AI offers remarkable potential, its implementation in drug discovery comes with challenges. One of the primary concerns is data quality and bias. Machine learning models are only as good as the data they are trained on, and if the datasets are biased or incomplete, AI predictions may be inaccurate or even harmful. Ensuring high-quality, diverse data is essential to prevent biased outcomes that could disproportionately affect certain patient populations.
Another concern is the "black box" nature of some AI algorithms. While AI can make accurate predictions, the reasoning behind these predictions is not always transparent, which can be problematic in highly regulated industries like pharmaceuticals, where understanding the mechanisms behind decisions is crucial for regulatory approval.
The Future of AI in Drug Discovery: Quantum Computing and Beyond
As we look to the future, integrating AI with other advanced technologies like quantum computing promises to further accelerate drug discovery. Quantum computing, still in its infancy, has the potential to perform complex calculations at unprecedented speeds, unlocking new possibilities for molecular simulations and chemical reactions that are currently beyond the capabilities of classical computers. AI could harness this computational power to solve some of the most intractable problems in drug discovery, such as predicting protein folding—a critical aspect of understanding how drugs interact with their targets.
Conclusion: AI as a Catalyst for Innovation in Drug Discovery
AI and machine learning are driving a paradigm shift in how drugs are discovered, developed, and brought to market. From target identification to clinical trials, AI is speeding up every phase of the drug discovery process, reducing costs, and increasing the precision of predictions. As AI technologies continue to evolve, their integration with other cutting-edge tools like quantum computing and CRISPR will open new frontiers in drug discovery, offering hope for more effective and personalized treatments.
In 2024 and beyond, AI will remain a central catalyst for innovation in drug discovery, reshaping the pharmaceutical landscape and bringing life-saving treatments to patients faster than ever before.
Comments